Deep Learning's Transformative Impact on Biomedical Engineering: Revolutionizing Healthcare
- Shuvranil Sanyal
- Sep 3, 2023
- 6 min read
Updated: Sep 4, 2023
Biomedical engineering stands at the intersection of engineering, biology, and medicine, aiming to develop innovative solutions to improve healthcare and advance our understanding of the human body. In recent years, deep learning has emerged as a transformative tool for enhancing research in biomedical engineering. Deep learning, a subset of machine learning, has the unique ability to autonomously learn and extract intricate patterns from vast datasets, making it well-suited for a wide range of applications in this dynamic and critical field. This blog aims at exploring the uses of deep learning in biomedical engineering research and eludes how it has revolutionized the approach to various problems in this domain.
1. Medical Imaging and Diagnosis
Deep learning has made remarkable strides in the field of medical imaging, particularly in tasks such as image segmentation, object detection, and disease diagnosis. Convolutional Neural Networks (CNNs), a type of deep learning model, have demonstrated exceptional performance in detecting tumors in medical images, classifying skin lesions, and identifying abnormalities in X-rays and MRI scans. These models can analyze intricate visual data with remarkable accuracy, greatly aiding healthcare professionals in early disease detection and treatment planning.
For example, in cancer research, deep learning models have been employed to identify cancerous regions in histopathological slides, significantly reducing the workload for pathologists and potentially improving diagnostic accuracy. Such applications of deep learning have the potential to revolutionize disease detection and monitoring, ultimately leading to better patient outcomes.
2. Drug Discovery and Development
Biomedical engineers often work on drug discovery and development projects, where the identification of potential drug candidates is a complex and time-consuming process. Deep learning has played a pivotal role in accelerating this process by predicting molecular properties, simulating drug-protein interactions, and designing novel molecules with desired properties.
Generative models like Generative Adversarial Networks (GANs) and Variational Autoencoders (VAEs) have been harnessed to generate molecular structures and explore chemical space more efficiently. This innovative approach has led to the discovery of new drug candidates and the optimization of existing ones, ultimately speeding up the drug development pipeline and bringing life-saving medications to patients more quickly.
3. Predictive Modeling and Personalized Medicine
Personalized medicine, a paradigm shift in healthcare, aims to tailor medical treatments to individual patients based on their genetic makeup, lifestyle, and other factors. Deep learning models can analyze vast amounts of patient data, including genomics, electronic health records, and medical images, to develop predictive models for disease risk, treatment response, and patient outcomes.
These models can assist clinicians in making informed decisions and recommending personalized treatment plans. For instance, predicting a patient's likelihood of developing complications after surgery or their response to a specific medication can significantly improve patient care and outcomes, reducing adverse events and treatment costs.
4. Brain-Computer Interfaces (BCIs) and Neuroengineering
In the field of neuroengineering, deep learning has played a pivotal role in developing Brain-Computer Interfaces (BCIs) that enable direct communication between the human brain and external devices. Deep learning algorithms can decode neural signals recorded from electrodes implanted in the brain, allowing paralyzed individuals to control prosthetic limbs or communicate through thought alone.
BCIs powered by deep learning have shown tremendous promise in restoring mobility and independence to individuals with spinal cord injuries and neurological disorders. The ability to translate neural activity into actionable commands has the potential to transform the lives of countless patients and open new avenues for rehabilitation and neuroscientific research.
5. Bioinformatics and Genomic Analysis
The field of bioinformatics relies heavily on deep learning for tasks such as DNA sequence analysis, protein structure prediction, and functional genomics. Deep learning models can extract meaningful information from the vast amount of genomic and proteomic data generated through high-throughput sequencing and other experimental techniques.
By identifying genetic variations associated with diseases, predicting protein structures, and uncovering gene regulatory networks, deep learning contributes significantly to our understanding of biology and disease mechanisms. This knowledge can guide the development of targeted therapies and precision medicine approaches, ushering in an era of more effective and personalized healthcare.
6. Natural Language Processing (NLP) in Biomedical Literature
Deep learning techniques, particularly in the domain of NLP, have been applied to the vast amount of biomedical literature available in the form of research articles, clinical notes, and patient records. These methods help extract valuable insights, identify relevant information, and streamline literature reviews for researchers and healthcare practitioners.
Sentiment analysis, topic modeling, and information extraction from text have all been enhanced by deep learning algorithms. This not only accelerates the research process but also assists in staying up-to-date with the latest advancements in the field, facilitating evidence-based decision-making.
7. Drug Repurposing and Adverse Event Detection
Deep learning has become instrumental in drug repurposing efforts, where existing drugs are investigated for new therapeutic uses. By analyzing molecular structures, biological pathways, and clinical data, deep learning models can identify potential drug candidates for repurposing, potentially saving time and resources in drug development.
Furthermore, deep learning models can monitor adverse events and drug interactions by analyzing electronic health records and pharmacovigilance data. Early detection of adverse events can lead to the timely withdrawal of unsafe drugs from the market and improved patient safety.
8. Personal Health Monitoring and Wearable Devices
The advent of wearable devices and the Internet of Things (IoT) has generated vast streams of personal health data. Deep learning algorithms can process and analyze this data to provide valuable insights into an individual's health and well-being. These insights can range from monitoring vital signs, predicting disease onset, and suggesting lifestyle modifications to improve overall health.
Wearable devices equipped with deep learning capabilities can continuously collect data on a person's activity, heart rate, sleep patterns, and more. This information can be used to create personalized health recommendations and early warning systems for conditions such as atrial fibrillation, sleep apnea, and diabetes.
9. Ethical Considerations and Data Privacy
While deep learning offers immense potential in biomedical engineering research, it also raises ethical concerns and data privacy issues. The use of personal health data, genetic information, and medical records must adhere to strict ethical guidelines and privacy regulations. Protecting patient confidentiality and ensuring informed consent are paramount in conducting responsible research in this field.
Furthermore, the potential for bias in deep learning models, particularly in healthcare applications, is a significant concern. Biased algorithms can lead to disparities in healthcare outcomes, particularly for underrepresented populations. Researchers and engineers must actively address bias through data preprocessing, model selection, and ongoing monitoring.
In other words, Deep learning has become an indispensable tool for enhancing research in biomedical engineering. Its capacity to analyze complex data, make accurate predictions, and automate tasks has fundamentally transformed the way researchers approach problems in this domain. From improving medical imaging and diagnosis to accelerating drug discovery, advancing personalized medicine, enabling BCIs, and unraveling the mysteries of genomics, deep learning is driving innovation and improving healthcare outcomes.
As the field continues to evolve, interdisciplinary collaboration between biomedical engineers, data scientists, clinicians, and ethicists will be essential to harness the full potential of deep learning. Together, they can address pressing medical challenges, ensure ethical and responsible use of data, and improve the quality of life for patients worldwide.
10. References
D.R. Sarvamangala and R. V. Kulkarni, "Convolutional neural networks in medical image understanding: a survey", Evol Intell. 2022; 15(1): 1–22.
J. Lan, M. Chen, J. Wang, M. Du, Z. Wu, H. Zhang, Y. Xue, T. Wang, L. Chen, C. Xu, Z. Han, Z. Hu, Y. Zhou, X. Zhou, T. Tong, G. Chen, "Using less annotation workload to establish a pathological auxiliary diagnosis system for gastric cancer", Cell Rep Med. 2023 Apr 18; 4(4): 101004.
Q. Vanhaelan, Y.C. Lin, A. Zhavoronkov, "The Advent of Generative Chemistry", ACS Med Chem Lett. 2020 Aug 13; 11(8): 1496–1505.
S. J. MacEachern, N. D. Forket, "Machine learning for precision medicine", Genome. 2020 Oct 22; 64(4): 416-425.
X. ZHang, Z. Ma, H. Zheng, T. Li, K. Chen, X. Wang, C. Liu, L. Xu, X. Wu, D. Lin, H. Lin, "The combination of brain-computer interfaces and artificial intelligence: applications and challenges", Ann Transl Med. 2020 Jun; 8(11): 712.
S.N. Abdulkader, A. Atia, M. Mostafa, "Brain computer interfacing: Applications and challenges", Egyptian Informatics Journal. 2015 July; 16(2): 213-230.
S. Min, B. Lee, S. Yoon, "Deep learning in bioinformatics", Briefings in Bioinformatics, Volume 18, Issue 5, September 2017, Pages 851–869.
D. Grapov, J. Fahrmann, K. Wanichthanarak, S. Khoomrung, "Rise of Deep Learning for Genomic, Proteomic, and Metabolomic Data Integration in Precision Medicine", OMICS. 2018 Oct 1; 22(10): 630–636.
U. Naseem, A.G. Dunn, M. Khushi, J. Kim, "Benchmarking for biomedical natural language processing tasks with a domain specific ALBERT", BMC Bioinformatics, 23(144), 2022.
C.Y. Lee, Y.P. Chen, "Prediction of drug adverse events using deep learning in pharmaceutical discovery", Brief ings in Bioinformatics. 2021 Mar 22;22(2):1884-1901.
M. Javaid, A. Haleem, R. P. Singh, R. Suman, S. Rab, "Significance of machine learning in healthcare: Features, pillars and applications", International Journal of Intelligent Networks, Volume 3, 2022, Pages 58-73.
W. Wang, W. Hsu, "Integrating Artificial Intelligence and Wearable IoT System in Long-Term Care Environments", Sensors. 2023, 23(13), 5913.
S. Gerke, T. Minssen, G. Cohen, "Ethical and legal challenges of artificial intelligence-driven healthcare", Artificial Intelligence in Healthcare. 2020 : 295–336.
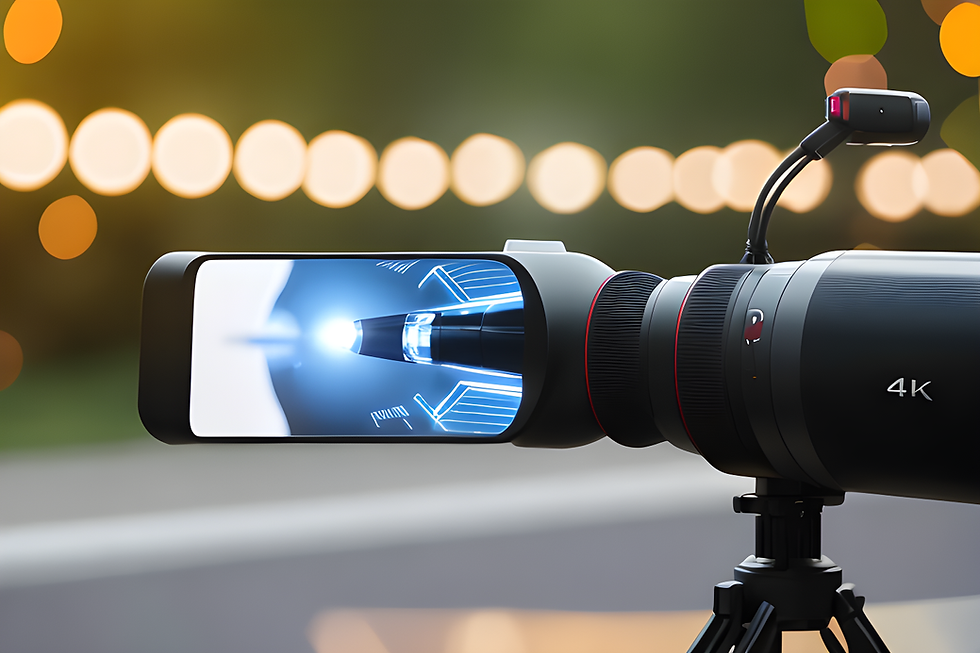
A very informative exposition